Harnessing Healthcare Datasets for Machine Learning: A Comprehensive Guide
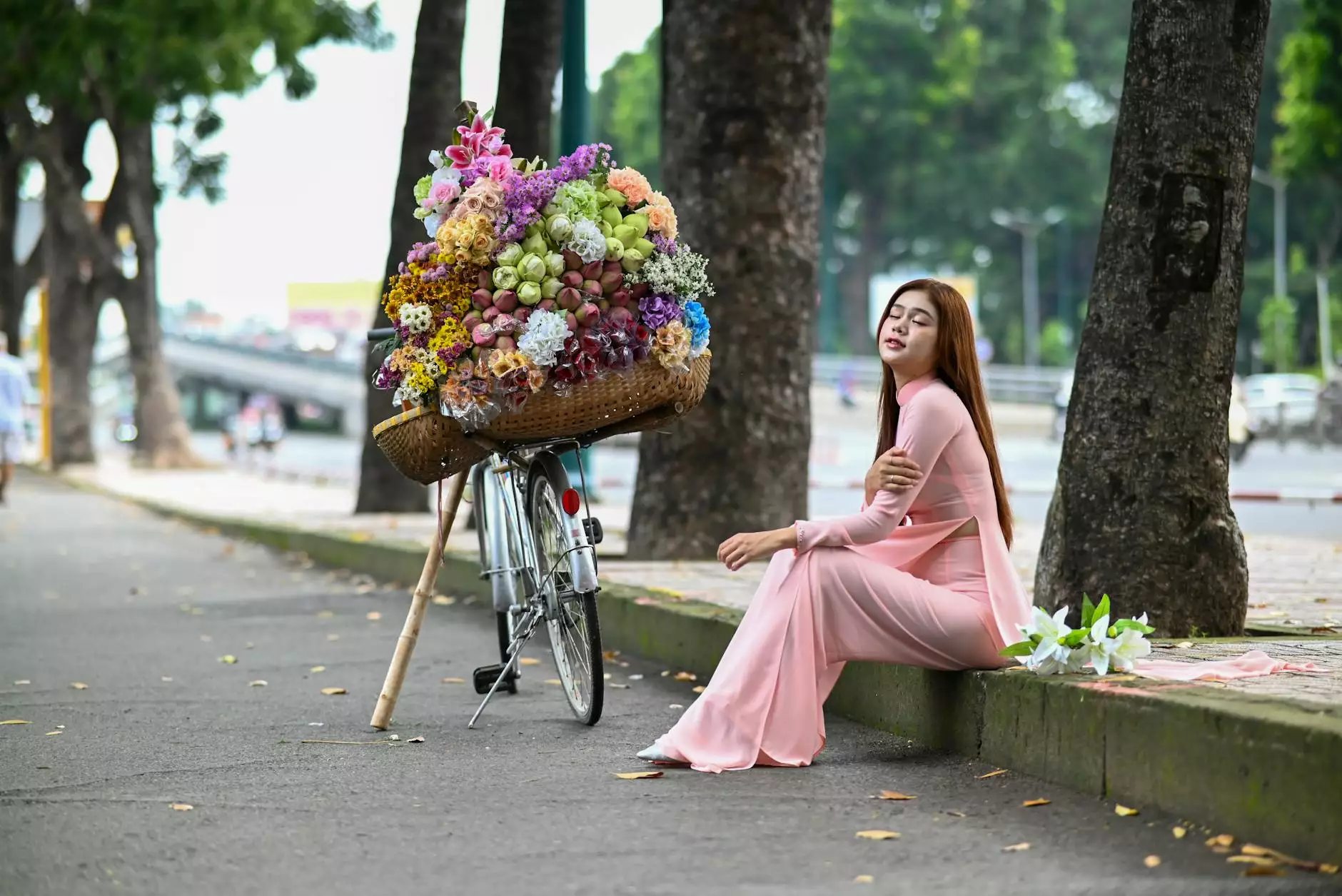
In today's digital age, data is permeating every aspect of our lives, and the healthcare sector is no exception. The use of healthcare datasets for machine learning has become an essential tool for improving patient outcomes, streamlining operations, and innovating services. This detailed guide will explore the importance of these datasets, their applications, the challenges faced in utilizing them, and how businesses can harness their potential for competitive advantage.
The Importance of Healthcare Datasets in Machine Learning
The integration of machine learning in healthcare is transforming the industry, making data more critical than ever. Here’s why healthcare datasets are so important:
- Predictive Analytics: Machine learning algorithms can analyze vast amounts of data to predict health trends, patient disease risks, and treatment efficacies.
- Improved Patient Care: By processing datasets, healthcare professionals can create personalized treatment plans that cater to individual patient needs.
- Operational Efficiency: Identifying inefficiencies in hospital operations through data analysis leads to lower costs and higher service levels.
- Research and Development: Healthcare datasets drive breakthroughs in drug development and medical research, contributing to more effective therapies.
Types of Healthcare Datasets
Healthcare datasets come in various forms, each serving different purposes. Understanding these can help businesses strategically implement machine learning solutions:
1. Electronic Health Records (EHRs)
Electronic health records are comprehensive digital versions of patients’ paper charts. They are critical for monitoring patient histories and treatment outcomes, providing vital data for analytics.
2. Clinical Trials Data
Data collected from clinical trials is invaluable for understanding the efficacy of new treatments and medications, offering insights into patient responses and overall effectiveness.
3. Administrative Data
Administrative datasets include billing information, patient demographics, and operational metrics, which are useful for improving business processes and healthcare delivery.
4. Health Surveys
Health surveys provide insight into public health trends, behaviors, and opinions, enabling policymakers and healthcare providers to adjust services accordingly.
5. Wearable Devices
With the rise of technology, data from wearable health devices is becoming increasingly available, offering real-time insights into patient activities and health status.
Applications of Machine Learning in Healthcare
Utilizing healthcare datasets for machine learning unlocks a variety of applications that can significantly enhance business operations and patient outcomes. Below are some of the key applications:
1. Disease Prediction and Management
Machine learning algorithms analyze historical patient data to predict risks of diseases such as diabetes or heart disease. By identifying at-risk individuals early on, healthcare providers can intervene before conditions worsen.
2. Personalized Medicine
Using datasets, healthcare professionals can tailor treatments to individual patients, ensuring they receive the most effective medications based on their unique genetic profiles.
3. Image Analysis
Machine learning facilitates advanced image analysis, allowing for early detection of diseases through radiology and pathology images. Algorithms can help identify anomalies that may be missed by the human eye.
4. Drug Discovery
Data analytics plays a critical role in drug discovery processes, enabling researchers to find suitable compounds more quickly, speeding up the time it takes for new treatments to reach the market.
Challenges in Utilizing Healthcare Datasets
While the benefits are significant, businesses face several challenges when it comes to utilizing healthcare datasets for machine learning:
- Data Quality: Poor data quality can lead to inaccurate predictions, making it essential to implement stringent data management practices.
- Privacy Concerns: Protecting patient data is paramount. Businesses must comply with regulations like HIPAA to ensure data is securely handled.
- Integration Issues: Many healthcare organizations have legacy systems that make integrating new machine learning solutions challenging.
- Data Silos: Data is often collected in separate systems, making it difficult to access and analyze comprehensive datasets effectively.
Best Practices for Leveraging Healthcare Datasets
To fully leverage healthcare datasets and maximize the benefits of machine learning, businesses should adhere to several best practices:
1. Prioritize Data Governance
Establishing a robust data governance framework ensures high data quality and compliance with regulations, facilitating trust in the data utilized for machine learning.
2. Invest in Analytics Tools
Implementing advanced analytics tools can help organizations effectively manage and analyze large datasets, enabling more precise insights and applications of machine learning.
3. Foster Collaboration
Encouraging collaboration between data scientists, healthcare professionals, and IT departments can generate innovative solutions and overcome integration challenges.
4. Continuous Learning and Adaptation
The healthcare landscape is continuously evolving, so it’s vital for organizations to stay updated on the latest machine learning technologies and adapt their strategies accordingly.
The Future of Healthcare and Machine Learning
The future of healthcare is undeniably intertwined with the advancements in machine learning and data analytics. As businesses increasingly adopt healthcare datasets for machine learning, we can expect numerous transformations:
1. Enhanced Predictive Models
With improved algorithms and more comprehensive datasets, predictive analytics will become more accurate, helping providers anticipate health issues before they arise effectively.
2. Broader Adoption of Telemedicine
The use of machine learning will further enhance telemedicine services, expanding access to healthcare professionals and supporting remote monitoring of patients.
3. Integration of AI in Clinical Workflows
AI-driven tools will likely be integrated into clinical workflows, streamlining processes and significantly reducing the administrative burden on healthcare providers.
4. Improved Population Health Management
Data-driven insights will enable healthcare organizations to develop more effective public health strategies, targeting specific populations and reducing health disparities.
Conclusion
The use of healthcare datasets for machine learning is shaping the future of the healthcare industry, offering unprecedented opportunities for improving patient care and operational efficiency. By understanding the types of datasets available, the applications of machine learning, and the challenges faced, businesses can strategically position themselves to harness these valuable resources. Looking ahead, the integration of advanced analytics in healthcare will be crucial in overcoming current challenges and driving innovation, ultimately leading to better health outcomes and enhanced patient experiences.